HOLISTIC VISUAL DATA REPRESENTATION FOR BUILT ENVIRONMENT ASSESSMENT
Price
Free (open access)
Volume
Volume 13 (2018), Issue 4
Pages
11
Page Range
516 - 527
Paper DOI
10.2495/SDP-V13-N4-516-527
Copyright
WIT Press
Author(s)
JUNG MIN HAN & NAMJU LEE
Abstract
With an increasing interest of big data and its analysis, urban planners and architects use manifold data from different sources as an indicator of urban phenomena. To analyse them, machine learning models have been gotten fame to evaluate complex urban features with correlation matrix and cross validation. There are two major types of data such as top-down and bottom-up data. Interestingly, there is a third category of data that has not been widely deployed yet which we define as the ‘Holistic visual data’. It is the general impression to your visual perception of urban environment when you at a specific spot that we believe can be captured by Google Street Views. This paper aims to ask how do top-down, bottom- up, and holistic visual data work individually or together to predict the built environment value in urban areas. Admittedly, housing price is a highly spatial metric that involves multifarious interests including macroeconomic policies, the development of the area, the local people, and specific houses’ features. To investigate the quality of built environment, machine learning techniques are adopted for different groups of datasets. By comparing several regression and classification models for each groups of data such as top-down, bottom-up and holistic visual data with housing price, the Random Forest model could be proposed as a best model. The intricate urban matrix needs to be organized such an order, but there are multiple factors affecting urban issues including land values and housing prices. By utilizing relevant machine learning models, it can be provided the practical guideline to architects and designers for improving the quality of built environment.
Keywords
bottom-up data, classification, data science, feature selection, holistic visual data, housing price prediction, machine learning, random forest regressor, top-down data, urban analysis
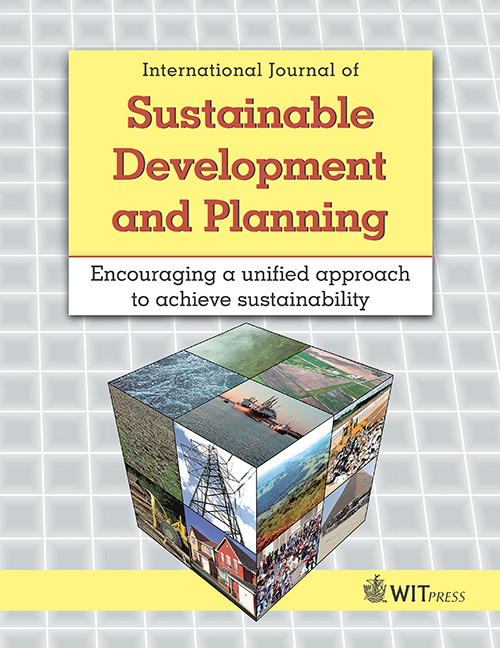