Using lagged and forecast climate indices with artificial intelligence to predict monthly rainfall in the Brisbane catchment, Queensland, Australia
Price
Free (open access)
Volume
Volume 10 (2015), Issue 1
Pages
12
Page Range
29 - 41
Paper DOI
10.2495/SDP-V10-N1-29-41
Copyright
WIT Press
Author(s)
J. ABBOT & J. MAROHASY
Abstract
Brisbane, the capital of Queensland, Australia, has flooded periodically and catastrophically, most recently in January 2011. Official seasonal rainfall forecasts failed to predict the floods. Since winter 2013, the Australian Bureau of Meteorology uses a general circulation model, the Predictive Ocean Atmosphere Model for Australia (POAMA), to make official seasonal rainfall forecasts presented as the conditional probability of rainfall being greater or less than the long-term median rainfall. We show that a more skilful forecast can be made using an artificial neural network (ANN), a form of statistical modelling based on artificial intelligence. A Jordan recurrent neural network with one hidden layer was implemented, using genetic optimization of inputs. For the sites of Gatton and Harrisville, in the Brisbane catchment, monthly rainfall forecasts from the ANN show lower root mean square errors than forecasts from POAMA. These rainfall forecasts from the ANN model were further improved by using inputs of independently forecast values for climate indices including the Southern Oscillation Index, the Interdecadal Pacific Oscillation, Pacific sea surface temperature anomalies (Niño 3.4) and also atmospheric temperature. The results presented here represent a first attempt at independently forecasting climate indices using an ANN model for the Australian east coast.
Keywords
Rainfall, forecast, lagged climate indices, SOI, IPO, Niño 3.4, Brisbane, flood, artificial neural network.
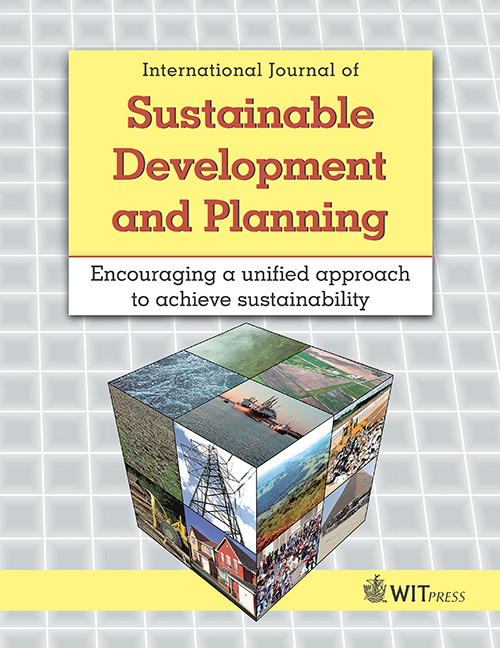