CLUSTERING APPROACH APPLIED ON AN ARTIFICIAL NEURAL NETWORK MODEL TO PREDICT PM10 IN MEGA CITIES OF MEXICO
Price
Free (open access)
Volume
Volume 11 (2016), Issue 4
Pages
11
Page Range
566 - 577
Paper DOI
10.2495/SDP-V11-N4-566-577
Copyright
WIT Press
Author(s)
E. MAGAÑA-VILLEGAS, J.M. CARRERA-VELUETA, S. RAMOS-HERRERA, J.R. HERNÁNDEZ-BARAJAS, C. GONZÁLEZ-FIGUEREDO, J.R. LAINES-CANEPA, A. VALDÉS-MANZANILLA & R.G. BAUTISTA-MARGULIS
Abstract
A cluster-based artificial neural network model called CLASO (Classification-Assemblage-Association) has been proposed to predict the maximum of the 24-h moving average of PM10 concentration on the next day in the three largest metropolitan areas of Mexico. The model is a self-organised, real-time learning neural network, which builds its topology via a process of pattern classification by using an historical database. This process is based on a supervised clustering technique, assigning a class to each centroid of the hidden layer, employing the Euclidean distance as a hierarchical criterion. A set of ARIMA models was compared with CLASO model in the forecast performance of the 24-h average PM10 concentration on the next day. In general, CLASO model produced more accurate predictions of the maximum of the 24-h moving average of PM10 concentration than the ARIMA models, although the latter showed a minor tendency to underpredict the results. The CLASO model solely requires to be built a historical database of the air quality parameter, an initial radius of classification and the learning factor. CLASO has demonstrated acceptable predictions of 24-h average PM10 concentration by using exclusively regressive PM10 concentrations. The forecasting capabilities of the model were found to be satisfactory compared to the classical models, demonstrating its potential application to the other major pollutants used in the Mexican air quality index.
Keywords
air quality, artificial neural network, clustering, PM10 modelling
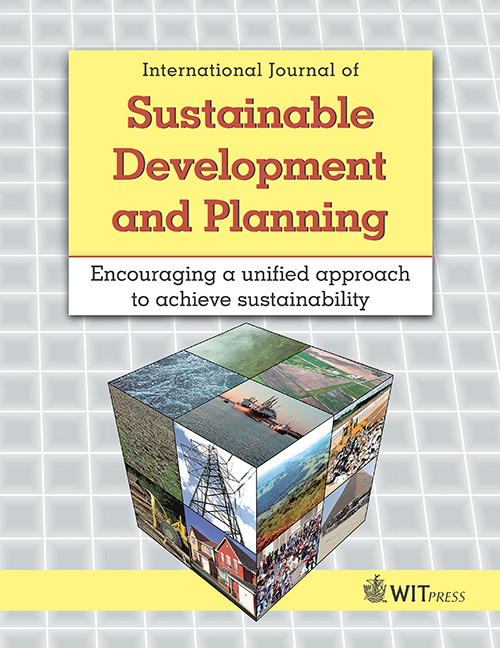